One of the more difficult challenges for modeling is deciding how (or if) to deal with extreme data points. It’s a common problem in economic and financial numbers. Fat tailed distributions are standard fare in stock market returns, for example. Meanwhile, the dramatic collapse in the economy during the pandemic last year is a reminder that outliers pop up in macro analytics too.
That leads to the question: What to do? There are many answers, which vary depending on the data set and the analytical goals. Quite often the issue arises in regression analysis, the foundation for many modeling applications. One solution is to ditch the standard linear regression and replace it with quantile regression, which is less vulnerable to extreme data points.
What’s the difference in these regressions? Without going too far down the rabbit hole, linear regression (LR) uses a least squares methodology to calculate the conditional mean to model the data. Quantile regression (QR) estimates the conditional median (or any other quantile, or quantiles, you select). That gives QR several advantages over LR, including a higher degree of stability when confronting outliers.
As a simple example, consider two sets of numbers – Data A and Data B. These are relatively well-behaved data sets and so there’s a relatively clear, tight relationship. As a result, running linear and quantile regression yields similar results. (Note: the QR model uses the median quantile in this example.)
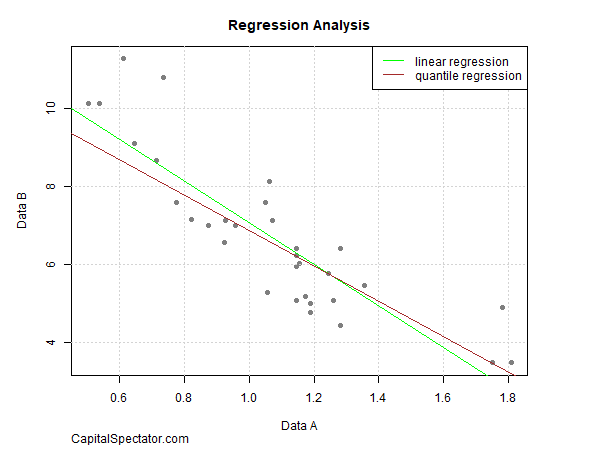
But it’s another story when we add some outlier data points, shown in red in the next chart below. Re-running the regressions that include the outliers shows that LR results shift substantially — indicated by the dashed green line (LR with outlier data) vs. the solid green line (LR without outlier data). By comparison, the two quantile regressions are essentially unchanged, which indicates that the outliers have little impact on the results. (Yes, the two QR regression lines are hard to see because they’re essentially identical.)
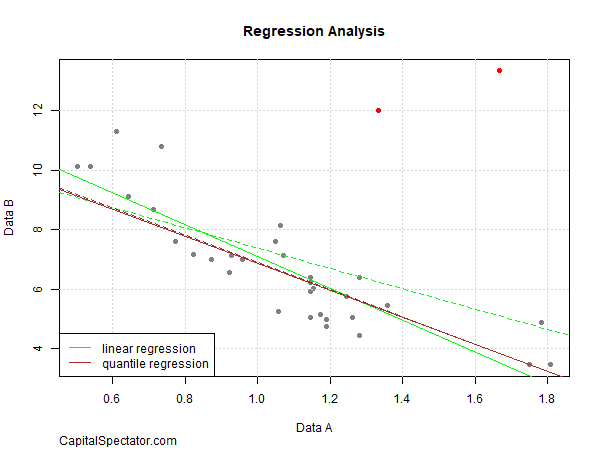
The more robust results in QR represent a powerful way to keep extreme events from creating havoc in financial and economic modeling efforts. That’s an especially useful tool in a world where fat tails feature in economic and financial data distributions.
Another advantage of QR, which I’ll detail in an upcoming post, is the ability to compute regressions at various quantiles, which provides a broader, more flexible profiling application with modeling.
There are still cases when using a basic linear regression makes sense. The good news is that there are alternatives, and quantile regression is on the short list.